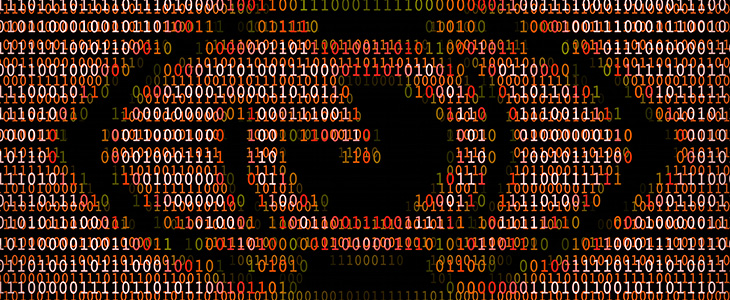
It is no secret that AML compliance is being revolutionized by the use of modern analytical and data management tools. At the heart of this enormous shift away from manually-intensive and strictly rules-based processes are the suite of artificial intelligence technologies, with their ability to analyze vast amounts of structured and unstructured data, detect linkages and patterns, and automate workflow. And whether you deem them the chicken or the egg, federal and state AML supervisors increasingly are reflecting in rule proposals and enforcement actions their expectation that such advanced methodologies will be used in satisfying existing and new regulatory requirements.
The tipping point for the use of new technology also has arrived regarding the management of insider trading, market manipulation and other suspicious market activities. These are all problems that have vexed the financial industry for decades. These crimes are also money laundering concerns because they involve illicitly derived funds. Detecting illegal market activities is vital to preventing our financial systems from being misused and ensuring their integrity.
Traditional Digital Surveillance
Law enforcement uses various methods to uncover financial crimes, including wiretaps, phone records, trading logs and whistleblowers. In addition, a fundamental tool is evidence derived from emails, texts, chat room conversations, etc. (together, “emails”). Monitoring employee communications has been a fact of life in corporate America for many years. As Michael Scott of the TV series, The Office, admitted in a 2008 episode, “There are certain things a boss does not share with his employees. His salary, that would depress them…And I am not going to tell them that I will be reading their emails.”
Traditional digital surveillance is focused on content. A company monitoring its employees takes all the communications on its servers, scrubs out the attachments, and then applies rules against the text of each message. For example, a scan of employee emails looks for certain words (e.g., “embezzle,” “cover-up,” or “write-off”) or phrases (e.g., “off-the-books,” “no one will find out,” or “I’ll take care of it”) from a list of a couple of hundred or so keywords and phrases that hint at bad motivation or behavior. When the software finds them, the appropriate risk, compliance, or information security employees are alerted and they then review the messages.
The problems with this approach are myriad. They include:
- The enormous volume of unstructured data to be reviewed (emails can number in the millions each day for a large, global company)
- The distraction of reviewing redundancies in email streams and irrelevant data such as signatures and disclaimers
- The large number of false positives produced
- The crude nature of the analysis involved
- The propensity for bad actors to avoid or obfuscate incriminating language
- The inability to correlate behavior patterns
- The amount of critical information that is often in screened-out attachments or exchanged via other communication channels
A well-known example of email examination occurred in 2003 after the Federal Energy Regulatory Commission posted on its website approximately 1.5 million internal Enron messages, including those of the company's top executives. Most messages were sent from 1999 to 2001, a period when Enron executives were manipulating financial data, making false public statements and engaging in insider trading. A number of computer scientists mined the Enron email data. Significantly, this was done without reading content. They reported having gleaned significant information by tracking email routing and word usage patterns. For example, they identified the key persons involved in the scandal (which included some junior-level executives) by analyzing the patterns of who emailed whom and when, and whether these persons began changing their email communications after Enron went under investigation.
The Advent of New Technology
Over a decade later, a further inflection point arrived with the growth of technologies that greatly facilitated the organization of data and the detection of patterns. Of particular note for email surveillance is the combined use of natural language processing (NLP) and graph analytics. NLP is a means by which computers study, comprehend and derive meaning from human language in a smart and useful way. NLP has been used for many years to uncover complex patterns and anomalies in large quantities of text. Graph analytics is concerned with understanding structures in networks. It is a method of mapping and exploring relationships between individuals that is behind prompts such as “Do you know this person?” and “Would you like to connect?” Modern computing allows for graph analytics techniques to be applied to increasingly large data sets.
Graph Analytics
Graph analytics is tailor-made for detecting relationships between words and individuals. It has proven to be a powerful tool in the investigation of terrorist organizations, helping to pinpoint individuals not previously known or outside the network who are, in fact, persons of significance. Another example is its use in uncovering traders and brokers across branches and firms who have colluded together over a period of time.
The graph analytics process, which can be used as a large database, can take into account and synthesize countless factors. For example, in a non-financial context, if a baseball team’s manager is concerned about how to pitch to a slugger on an opposing team, he can use graph analytics (assuming a sufficient data set) to map out and identify the types, speeds and locations of pitches that were most successful over the hitter’s playing lifetime in keeping him off base, considering other relevant factors such as lefty versus righty pitcher, men on base and intentional walks.
Graph analytics has been used for many years in analyzing web pages. One example is the famous PageRank link analysis algorithm used by Google Search to rank websites in its search engine results. However, in the web page arena, you have the benefit of being able to take into account links between pages in addition to content. Such explicit links are not available for emails.
Regarding emails, the graph analytics platform sets up a baseline network and then maps the email data into objects (or nodes) and the connections (such as who wrote the email, to whom it was sent, and who else received it) among these objects. Other information subsets can then be layered in, including the role and significance of the senders and receivers, whether the email was sent outside the company, timing (e.g., late/odd hours, around dates of key financial developments), directionality (e.g., is one party doing most or all of the sending?), and frequency. Rules also can be applied to reduce the amount of messages that have to be screened (e.g., company-wide distributed emails are probably not a concern).
Natural Language Processing
Once the baseline graph is sufficiently refined, NLP can bring in the element of content. NLP, in addition to searching for keywords (e.g., “confidential,” “disclosure date,” or “target company”) and analyzing emotional status, can examine relationships between words and concepts within and across documents. “Lingo clustering” in particular can identify themes of potential concern in email threads, such as ones that involve a call to action with an underlying time urgency outside the scope of an employee’s typical work activities.
The combination of graph analytics and NLP allows for the pattern detection and anomaly identification within a huge amount of unstructured data, discerning relationships among customers, employees, companies, and transactions in a far more precise manner than previously possible.
For example, the graph analytics process can reveal that Person A, a senior company official who has access to confidential data regarding mergers and acquisitions, sends emails frequently to Person B (someone outside the company). Person B never responds. No other person is ever copied on the emails. The emails typically contain the words “price,” “sweet,” “credit,” and “pork.” They also contain words that have no obvious meaning and may be used as codes.
This situation contains many red flags and might initially be ranked as high risk. Thus, further analysis is performed. If it is found that Person B is a takeout Chinese restaurant to which many others in the company also send emails, the alert will likely be dropped. However, if Person B is a natural person, further information will be sought such as whether he or she is linked to another financial business, whether the emails are sent on or around key financial disclosure dates, and whether other senior officials in the company (particularly officials in non-business functions such as compliance) send or receive emails to or from the person.
If the emails from Person A continue to be viewed as high risk, NLP can analyze content, after initially performing “data cleaning” such as eliminating punctuations, converting to lower case, removing proper nouns, disregarding stop words or numbers, and reducing words to their roots. NLP then might search for clusters of keywords in numerous related documents that suggest an unlawful motive (e.g., “problem,” “quiet,” “compliance,” and “urgent”) and how they are sequenced. The ultimate goal is to find themes that point to illicit activity and to flag the emails involved.
Use for Traditional AML Compliance
NLP is an obvious tool for enhancing customer due diligence efforts, particularly given its ability to comprehensively “read” a vast amount of data and both identify correlations and links between events and people and to analyze sentiment. This allows for suspicious activity to be flagged in a manner that lessens the occurrence of false positives.
Graph analytics is a powerful tool in meeting the new beneficial ownership requirements. An entire network infrastructure and all its links can be represented in graphs, simplifying the process of understanding and tracing complex organizational structures. Graph analytics also can be used to determine relationship among AML documents.
The use of modern analytical and digital techniques such as graph analytics and NLP does not signal the end of an existing monitoring and surveillance infrastructure. Rather, they can feed into existing, traditional rule-based processes, by being used beforehand to eliminate a great deal of the noise and allow the surveillance process to be much more proficient. The bottom line is that the productivity and efficiency needed to satisfy the suite of modern AML requirements must now come from a combination of human and technological resources.