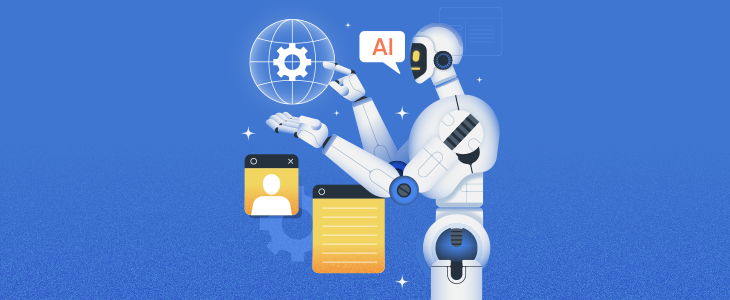
Suspicious activity report (SAR) filings are increasing. Last year, a whopping 4.6 million SARs and 20.8 million currency transaction reports (CTRs) were filed, according to the Financial Crimes Enforcement Network’s (FinCEN) Year in Review FY 2023.1 That is nearly a 28%2 increase in year-over-year SAR filings. The sheer volume of SAR filings alone can easily overwhelm financial crime compliance organizations.
Why so many SAR filings? Banks and financial institutions (FIs) consider “suspicious” a low bar to jump. This conservative approach makes transaction monitoring (TM) a challenging compliance obligation, as organizations must manually review millions of TM alerts each month, with most of those alerts being non-suspicious or false positives. These programs are typically the biggest compliance cost center, taking extensive time, requiring large teams of people and being rife with manual work and errors.
On June 28, 2024, FinCEN issued a proposed rule3 to strengthen and modernize FIs’ anti-money laundering/counter-terrorist financing (AML/CTF) programs. As part of the announcement, FinCEN stated, “The proposed rule would encourage FIs to modernize their AML/CTF programs where appropriate to responsibly innovate, while still managing illicit finance risks.”
Fortunately, artificial intelligence (AI) is becoming a game-changing innovation for AML/CTF programs. It can particularly help organizations more effectively manage SARs by providing better controls, increasing the consistency of approach to procedures, improving the quality of alert reviews, reducing material/immaterial errors and helping transform TM analysts from authors to editors. In addition, AI can provide a 30,000-foot view to identify patterns and links much more easily.
Automating Manual, Error-Prone Investigation Processes
Instead of spending time in analytical work, TM investigators spend most of their time doing hunter-gatherer work, spending a large part of their day gathering data and information and documenting such work rather than leveraging their true talent for fighting financial crime and managing and mitigating risk. Between 80% to 85% of the work analysts perform is spent tracking down information and evidencing their case review. With so many places to gather information, from various systems to relationship managers who can provide information, TM analysts are doing a lot of different tasks just to pull together everything before they can even analyze an alert. From there, they need to identify the information that is required to support an investigation. As a result, TM analysts are doing a lot of time-consuming and laborious work as part of an alert—even for a simple alert.
These manual, redundant processes can lead to errors. For example, let us say that the analyst had to sample five counterparties, and they did four by accident. The next thing, an auditor, quality assurance (QA), quality control (QC) or a regulator comes in and asks, “Why did you only do it four times?” They do not know why. It was purely a simple mistake, but a mistake, nonetheless.
By automating the tedious, error-prone work, including getting the information on the potential suspects and auto-populating the SAR narrative, AI drastically reduces mistakes, ensures complete information and frees up the analysts to work on higher-value/higher-risk type of work—turning analysts from authors to editors. Graphic 1 below details the workload associated with transaction alerts.
Graphic 1: Manual steps for an analyst to file a SAR
Source: WorkFusion
Avoiding the 30-Day Scramble
Organizations trying to determine if an alert needs to be escalated further and if they need to file a SAR typically work on a 30/30/30 schedule. This means it takes 30 days to review an alert; 30 days to work an alert that has been escalated to a case; and 30 days to file a SAR once a decision to file has been made. Many banks that work on this 90-day cycle, especially those that generate a lot of alerts, may not have the manpower to do the work and end up with backlogs.
But again, most of it will be a very manual process of reviewing the alert, pulling together the information that is necessary to comply with the procedures around that alert and the case, and then pulling all that information together for a narrative for the SAR, as well as filling out the SAR form that must get submitted to FinCEN.
Since AI can work around the clock and pick up and work alerts when generated, it takes care of the review immediately, helping avoid the timeline scramble.
Reducing the Nonsuspicious Noise
Many FIs still rely solely on traditional rule-based transaction monitoring systems. To ensure they are monitoring transactions as effectively and efficiently as possible, they cast a wide net of rules somewhat akin to using a sledgehammer on the fly, which generates high alert volumes and exceptionally high false-positive/not-suspicious rates. Of those alerts, 10% may get escalated to a case, and maybe 3-5% end up being a SAR, while only 1% is an actual financial crime.
For example, HSBC partnered with Google Cloud to create a transaction risk monitoring approach driven by AI, not rule-based scenarios. By utilizing the AI, HSBC says it was able to identify two to four times as much genuinely suspicious activity as the previous system while reducing the number of alerts generated by 60%. According to a Google Cloud blog,4 this afforded its investigations team more time to review genuinely suspicious cases and resulted in twice as much identified financial crime in its commercial banking operations and almost four times as much across retail banking.
Identifying Patterns
Let us take false positives/nonsuspicious alerts one step further; AI will also be able to give you a bird’s eye view into identifying patterns of behavior. AI will be able to review for and surface up for an analyst account linkages and patterns very quickly, whereas a human would either not make the connection due to the complex nature of the relationships or would take significant amounts of time to identify. Using a real-life experience as an example, while conducting an investigation where it was necessary to review approximately ten accounts of a customer, after reviewing the transaction activity, links were found to over 70 accounts. Although the accounts were identified, it took over a week’s time and construction of a rather complex spreadsheet to track and add notes. With AI, this would have taken significantly less time as the AI could have reviewed each account, surfaced any new accounts identified in the transactions, pulled client data and summarized it into a document format digestible to analysts.
The Benefits of AI for SAR Filings
AI and generative AI (GenAI) hold the promise to revolutionize investigations and SAR filings: alleviating inefficiencies and inconsistencies, improving poor narrative quality and providing complete information. AI will help with quality issues in SAR forms, such as cuts and pastes of narratives, errors in inputting suspect information and incorrect timeframes and dollar amounts. As a matter of fact, in Celent’s 2023 Financial Crime Survey,5 200 anti-financial crime (AFC) executives were asked where they see AI having the most impact within their organizations. Leading the pack by a wide margin was transaction monitoring, with 38% of votes, followed by 27% for fraud, 25% for know your customer and 10% for sanctions.
If your organization is ready to move forward with AI to modernize SAR efficiency, there are two buckets to consider: governance and guardrails. You need to bring in regulators and senior management early in the process and look at how your model risk management (MRM) will approach it and how the regulators will approach it with your FI. You can address this by testing, documenting and going through your MRM framework to make sure the model has gone through the scrutiny needed to be deployed correctly.
Next, look at what the quality assurance/quality control process looks like after going live into production. Report on the AI/machine learning/GenAI results, ensure you have analytics to measure its effectiveness and how it compares to your human analysts, and monitor for bias and model drift. Finally, ensure you have a maintenance plan.
We are at an inflection point with AI use in financial crime programs, like where the industry was 20 to 25 years ago when the first automated, rule-based transaction monitoring and fuzzy logic-based screening software were introduced and deployed. Much like then, many banks and FIs have either implemented and deployed AI and machine learning into their AFC programs or are evaluating how it can be used to build more effective and efficient programs. It is no longer a question of when; it is a question of how a bank or FI brings AI and machine learning into their AFC programs, and specifically their transaction monitoring and SAR processes. Many banks have already taken the step forward, and eventually, the use of AI/machine learning in transaction monitoring and SAR programs will be a must.
Art Mueller, vice president of financial crime, WorkFusion
- “Financial Crimes Enforcement Network (FinCEN) Year in Review for FY 2023,” FinCEN, https://www.fincen.gov/sites/default/files/shared/FinCEN_Infographic_Public_508FINAL_2024_June_7.pdf
- “SAR Stats,” FinCEN, https://www.fincen.gov/reports/sar-stats
- “FinCEN Issues Proposed Rule to Strengthen and Modernize Financial Institutions’ AML/CFT Programs,” FinCEN, June 28, 2024, https://www.fincen.gov/news/news-releases/fincen-issues-proposed-rule-strengthen-and-modernize-financial-institutions
- “Fighting money launderers with artificial intelligence at HSBC,” Google Cloud Blog, November 29, 2023, https://cloud.google.com/blog/topics/financial-services/how-hsbc-fights-money-launderers-with-artificial-intelligence
- Ian Watson, “Sticking With the Devil You Know,” CELENT, July 19, 2024, https://www.celent.com/insights/740790254