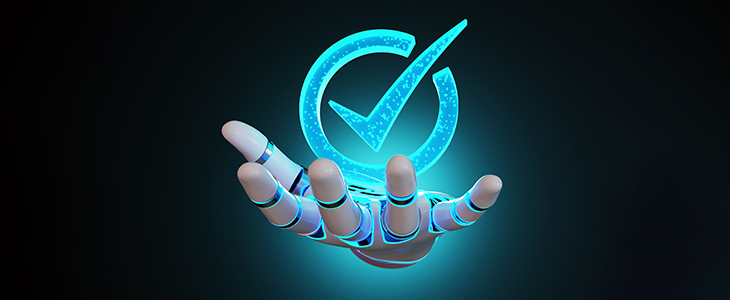
Nowadays, people often talk about artificial intelligence (AI) and machine learning. “Artificial Intelligence is the field of developing computers and robots that are capable of behaving in ways that both mimic and go beyond human capabilities. AI enabled programs can analyze and contextualize data to provide information or automatically trigger actions without human interference.”1 Simply, AI is the process by which computers and systems perform tasks in a similar manner as humans but with speed and decision-making skills that are defined by humans. Some of the examples in today’s world related to AI include smart devices, voice assistants (such as Siri), chatbots, translation applications, navigation/map apps, facial recognition tools, etc.
Machine learning is a pathway to AI. It is a subset of AI that utilizes “algorithms to automatically learn insights and recognize patterns from data, applying that learning to make increasingly better decisions.”2 Essentially, machine learning involves some rules and conditions within AI that learns from huge historical data sets for faster decision-making.
Organizations are involving AI and machine learning in their systems and strategic plans to take advantage of automating manual processes and act on data-driven insights with greater efficiency and effectiveness.
Financial institutions (FIs) utilize AI and machine learning technologies within financial crime compliance specifically for anti-money laundering/counter-terrorist financing (AML/CTF), sanctions and customer onboarding and take advantage of its benefits, which are described below.
AML/CTF
Regarding AML/CTF, the traditional method of transaction monitoring systems involves implementing detection scenarios based on certain parameters, logic and thresholds. This method is fading away, and FIs are focusing more on utilizing service providers that have AI and machine learning technologies integrated with their transaction monitoring systems and whose systems are not based only on detection scenario logic and threshold reviews but also employ smart technologies. Doing so goes beyond the traditional methods and ventures deeply into data analysis through various investigative transaction patterns for the alerted as well as historical transactions; analyzing customer profiles within the bank’s core banking systems; searching for information on social media platforms; and screening the customers from a financial crime perspective, link analysis, document processing, etc. In addition, AI/machine learning technologies are customizable, allowing organizations to pick and choose or add additional checks and have the flexibility to automatically close the related alerts as level 1 at a high pace with comprehensive customized narratives. The systems can be programmed to flag real cases as Level 2 so that reviewers can make necessary decisions accordingly. These technologies are continuously learning how to identify complex and unusual transactions. Some service providers even provide the flexibility to automatically close alerts at both level 1 and level 2, depending on organizational requirements.
Sanctions
Many FIs are facing the challenges of an enormous number of false positives for sanctions screening alerts related to customer screenings as well as payments screening. Payments are highly sensitive and time-bound transactions that must be processed within a defined turnaround time. We have seen banks being penalized for processing payments violating sanctions-related concerns. Basically, payments are highly sensitive as they are required to be reviewed properly to avoid any sanctions violations. At the same time, they need to be processed quickly due to their real-time nature. FIs have struggled a great deal to put the right parameters and screening logic in place.
Despite incorporating the higher end of thresholds, payment screening can result in a huge volume of false alerts that are not only time-consuming but delay the payment processing cycle as well. In order to have the effective and timely release of these payment screening alerts, some service providers are providing AI and machine learning-related advanced technologies that release bulk alerts with properly customized narratives in a short time as level 1 and refer potential real matches to level 2 reviewers for taking necessary actions. The alerts are reviewed by these models, analyzing the parameters and conditions that triggered the alert on all the involved parties in the transactions, referencing customer information, historical trends, results of in-depth Google searches and media searches, and adherence to the organization’s specific policies/requirements. The alerts are then either closed or escalated to the next level.
These AI models learn from historical data and evolving trends by improving detection over time. Again, the configurations are customizable depending on the requirements of the FIs. This gives the FIs the benefit of a solve rate on the match as well as the alert level and allows the compliance team to focus more on potential matches, thereby reducing the overall turnaround time for releasing the related alerts and spending focused time on other necessary sanctions activities.
Customer Onboarding
One more benefit to FIs that employ AI/machine learning capabilities is related to the customer onboarding journey. Some organizations have been using robotics process automation, which automates a number of workflow steps. However, using AI provides faster results for extremely large datasets for customer due diligence checks in terms of sanctions screening/negative media searches, Google searches, documentation checking and other related items. AI enables this to be done quickly with comprehensive narratives, providing ample time for level 2 reviewers to make informed decisions without delays and effectively manage their time.
The use of AI/machine learning technologies within AML/CTF, sanctions and customer onboarding provides comprehensive management information systems for proper hands-on analysis based on various identifiers.
Key advantages of utilizing AI/machine learning in financial crime compliance include faster processing, enormously increased data analysis and improvements in handling complex tasks, all of which result in effective decision-making that can help organizations properly manage their resources.
AI/machine learning can teach these models or tools to perform certain tasks and make decisions with predefined steps involving large datasets and algorithms. The more clarity there is in defining these models, the more effective the results that are achieved.
The future of financial crime compliance is AI, and technology is continuously evolving with advanced features. These tools must be used ethically and responsibly.
Ghaus Bin Ikram, CAMS, head of Financial Crime Compliance/MLRO, United Arab Bank PJSC, UAE,
Disclaimer: The views expressed are solely those of the author and are not meant to represent the opinions of his employer.
- “Artificial Intelligence (AI) vs. Machine Learning,” Columbia University, The Fu Foundation School of Engineering and Applied Science, https://ai.engineering.columbia.edu/ai-vs-machine-learning/
- Ibid.